The Impact of AI-Powered Medical Transcription on Clinical Documentation Practices
Feb 8, 2025
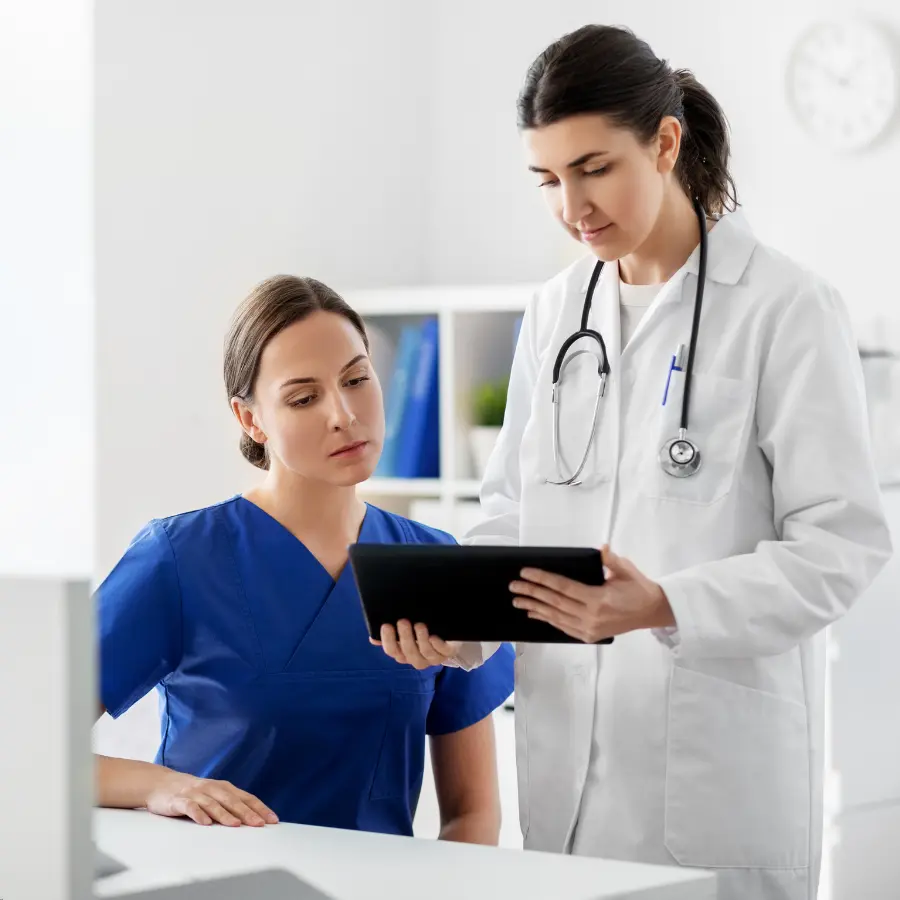
Medical transcription has historically been a critical component in the documentation of patient care, involving the conversion of voice-recorded reports as dictated by physicians and other healthcare providers into text format. Traditionally, this process required significant time and input from medical transcriptionists, who would listen to recordings and manually type them out. However, with the advent of AI transcription in healthcare, the landscape is undergoing a transformative shift. AI technologies are now being leveraged to automate and streamline the transcription process, thereby enhancing the accuracy and accessibility of medical records.
How AI Transcription Technology Works
AI in Clinical Documentation
This technology is particularly beneficial in medical settings where documentation accuracy is paramount. By analyzing vast amounts of audio data, AI systems can learn to recognize complex medical terminology and patient information, swiftly translating spoken words into precise clinical documentation. This process not only ensures a higher level of accuracy but also significantly expedites the documentation workflow, freeing medical professionals to focus more on patient care rather than paperwork.

The Role of Machine Learning
Machine learning (ML) is a core component and plays a pivotal role in refining the efficiency of medical transcription solutions. Here’s how ML contributes to enhancing transcription systems:
Continuous Learning: Unlike traditional speech recognition software, which follows predefined rules, ML algorithms evolve by analyzing voice data, user interactions, and manual corrections. The more data the system processes, the better it recognizes different accents, dialects, and medical terminology. This adaptability is particularly important in healthcare, where diverse linguistic patterns and specialized vocabularies exist. A system that initially struggles with regional pronunciations can gradually refine its accuracy by learning from repeated exposure to a specific physician’s voice. Continuous improvement reduces the need for manual corrections and enhances overall efficiency.
Contextual Understanding: Medical terminology often includes homophones—words that sound identical but have different meanings—making accurate transcription a challenge. ML-driven transcription software enhances contextual awareness by analyzing the surrounding words, sentence structure, and the specific medical context in which terms are used. For example, in a cardiology report, the system can differentiate between "hypertension" (high blood pressure) and "hypotension" (low blood pressure) based on the context of the discussion. This capability minimizes errors that could lead to serious misinterpretations of patient records. Also, ML models can recognize medical phrases that frequently appear together, ensuring that documentation maintains consistency and clarity.
Error Reduction: Maintaining the integrity of medical records requires a high degree of accuracy, and ML-powered transcription tools significantly reduce errors through automated correction mechanisms. These systems identify inconsistencies, flag potential transcription mistakes, and suggest corrections based on previously recorded patterns. If a physician frequently corrects a particular misinterpretation, the ML algorithm learns from this and proactively adjusts future transcriptions. AI-driven transcription can cross-check entries against standardized medical databases to identify discrepancies. It enhances reliability and reduces the risk of medical errors caused by documentation inaccuracies.
Customization: Every medical specialty has its own terminology, abbreviations, and documentation preferences, making a one-size-fits-all transcription solution impractical. ML-driven systems address this challenge by offering customizable features that cater to specific medical disciplines. A transcription system used in orthopedics can prioritize terms related to musculoskeletal conditions, while one used in oncology can recognize complex cancer-related vocabulary. Specialization allows for a higher level of accuracy tailored to the needs of different healthcare providers. Additionally, ML models can learn from individual physician preferences, adapting to commonly used phrases, preferred formatting styles, and specific shorthand expressions.
Digital medical transcription will become even more intelligent and capable as these technologies advance, allowing healthcare professionals to focus on patient care rather than administrative tasks. By embracing ML-driven transcription, the medical industry moves closer to a future where documentation is faster, more accurate, and seamlessly integrated into clinical workflows.
Real-Time Documentation
The real-time documentation capabilities of AI medical transcription platforms have become game-changers in healthcare. As doctors speak, the AI system transcribes their words instantly into the patient’s electronic health record (EHR), making the information available without delay. Immediacy ensures that patient records are always up-to-date, which is crucial during rapid decision-making situations such as emergency care. The ability to document interactions in real time also helps maintain a more comprehensive and accurate patient history.
Integrating AI Transcription With Electronic Health Records
Seamlessly integrating EHR systems is a critical advancement in modern clinical documentation. By automatically populating EHRs with transcribed data, this technology helps eliminate manual data entry errors and streamlines the workflow. This saves time and ensures that patient records are complete, consistent, and readily accessible to authorized healthcare providers. The result is a more efficient healthcare system with improved data management and patient care.
Benefits of AI Transcription in Healthcare
Reducing Administrative Burden
With AI handling the bulk of transcription duties, healthcare providers can redirect their time and efforts from administrative tasks to patient care. This shift improves productivity and enhances job satisfaction among clinicians who can focus more on clinical activities rather than paperwork. Moreover, faster documentation turnaround times mean that patient information is updated more swiftly, facilitating a smoother workflow and quicker patient throughput.
Cost Savings
The cost of AI medical transcription for transcription purposes also presents significant advantages. Automating the transcription process reduces the need for extensive documentation staff, cutting labor costs. Also, the enhanced speed and accuracy provided by AI reduce the expenses associated with corrections and delays that are common in manual processes. These savings can be reallocated to other critical areas within healthcare facilities, such as patient care or technological advancements, further improving the overall service quality.
Streamlining Patient Data Organization
Using automatic medical transcription tools helps organize patient data more effectively. By accurately capturing and categorizing information, AI systems facilitate easier access to patient histories, test results, and other critical data. Organization is crucial for healthcare providers when making informed decisions about patient care. Enhanced data management also supports more robust data analysis for research and quality improvement initiatives, potentially leading to better health outcomes and more personalized medicine approaches.

Challenges and Considerations in AI Medical Transcription
Data Privacy and Security Concerns
While AI in medical documentation offers numerous advantages, it also raises significant data privacy and security concerns. The following key areas highlight the major risks and necessary safeguards associated with medical AI scribes:
Cybersecurity Threats: Hackers may exploit vulnerabilities in these systems to steal or manipulate sensitive medical records, leading to severe consequences such as identity theft, insurance fraud, or compromised treatment plans. Common threats include ransomware attacks, phishing schemes, and insider breaches, all of which pose risks to the confidentiality and integrity of medical data. To mitigate these threats, healthcare organizations must adopt robust cybersecurity strategies, such as multi-factor authentication, endpoint protection, and intrusion detection systems.
Encryption & Access Controls: Encryption ensures that patient data remains unreadable to unauthorized users, even in the event of a security breach. Advanced encryption methods such as end-to-end encryption and secure socket layer (SSL) protocols can help safeguard data while it is being transmitted or stored. Implementing access controls such as role-based access permissions and biometric authentication prevents unauthorized individuals from accessing sensitive records.
AI Bias & Data Integrity: If an AI system is trained on incomplete or biased datasets, it may produce inaccurate documentation, leading to potential errors in patient care. For example, if an AI model does not have sufficient diversity in training data, it may struggle to document medical conditions in underrepresented populations accurately. Automated documentation systems may misinterpret medical jargon or context, leading to data integrity errors. AI systems should undergo continuous testing and refinement using diverse, high-quality datasets to mitigate these risks. Human oversight is also critical in reviewing AI-generated documentation to ensure accuracy.
By proactively implementing these measures, healthcare providers can harness the benefits of AI-driven documentation while maintaining trust, security, and compliance in the healthcare industry.
Ensuring Compliance With Regulatory Standards
Compliance with regulatory standards such as HIPAA is crucial in the deployment of automated medical transcription. These regulations are designed to safeguard patient information and ensure that it is handled appropriately. AI systems must be programmed to comply with these standards, which can be challenging given the complexities of AI technology and continuous updates. Regular audits and updates to AI systems are essential to maintaining compliance and protecting patient information effectively.
The Future of AI in Medical Documentation
Expanding Beyond Transcription
As AI technology matures, its role in healthcare is becoming more multifaceted and integral to various aspects of patient care and medical administration. Here’s how AI is expanding its footprint in the healthcare sector:
Predictive Analytics: By analyzing vast datasets - including patient histories, lab results, and social determinants of health - AI can identify patterns that may signal potential health risks. Predictive models can assess a patient’s likelihood of developing diabetes, cardiovascular disease, or infections, allowing for earlier interventions. AI also helps hospitals optimize resource allocation by forecasting patient admission rates and identifying potential surges in demand for medical services. This predictive capability enhances operational efficiency, reducing wait times and improving patient outcomes. AI models can also provide real-time alerts for conditions such as sepsis, enabling clinicians to act quickly before complications arise.
Diagnostic Support: AI is increasingly vital in diagnostic medicine, improving accuracy and reducing the time required for disease detection. By utilizing deep learning algorithms, AI can analyze medical images such as X-rays, MRIs, and CT scans with remarkable precision, identifying anomalies that human radiologists may overlook. In dermatology, AI-powered tools can assess skin lesions for early signs of melanoma, while in pathology, they assist in detecting cancer cells in biopsy samples. Beyond imaging, these diagnostics extend to genetic testing, where algorithms analyze DNA sequences to identify hereditary diseases or predict treatment responses. The speed at which AI processes vast amounts of diagnostic data enables quicker decision-making, allowing physicians to start treatments sooner.
Personalized Medicine: The era of one-size-fits-all treatment plans is gradually being replaced by personalized medicine, thanks to AI’s ability to analyze individual patient data. By integrating genetic profiles, lifestyle factors, and medical history, AI can recommend tailored treatment strategies that maximize effectiveness and minimize adverse effects. AI can help oncologists determine the most effective chemotherapy regimen based on a patient’s genetic markers, improving cancer treatment outcomes. In pharmacology, AI-driven models assist in predicting how individuals will metabolize medications, ensuring more precise dosing. Additionally, AI supports personalized nutrition and lifestyle interventions by analyzing real-time health data from wearable devices. Such an individualized approach enhances patient adherence to treatments and reduces trial-and-error prescribing.
Remote Patient Monitoring: Wearable devices, smart sensors, and AI-driven apps can track vital signs such as heart rate, oxygen levels, and blood pressure in real time, sending alerts to healthcare providers if abnormalities are detected. This technology is particularly beneficial for managing chronic conditions such as diabetes, hypertension, and heart disease, allowing physicians to intervene before complications arise. AI-enhanced RPM also improves elderly care by detecting falls, monitoring mobility, and assessing cognitive decline. Patients recovering from surgery can be monitored remotely, reducing unnecessary hospital visits and ensuring timely interventions. By extending healthcare beyond hospital walls, AI-powered remote monitoring enhances accessibility, reduces hospital readmissions, and empowers patients to take an active role in their health management.
With advancements in predictive analytics, diagnostic support, personalized medicine, and remote monitoring, medical scribe automation is helping healthcare providers make data-driven decisions that improve patient outcomes.
Enhances Collaboration Among Providers
The use of AI transcription solutions in healthcare facilities enhances collaboration among medical professionals by ensuring that accurate and up-to-date patient information is readily accessible. Shared access facilitates a more coordinated approach to patient care, where specialists and general practitioners can align their treatment plans based on comprehensive, real-time documentation. The collaborative environment supported by AI improves the continuity of care and helps form a holistic view of the patient's health, leading to better treatment outcomes.

Preparing for Widespread Adoption
This preparation involves training medical staff to work effectively with AI tools, upgrading IT infrastructure to support advanced AI applications, and developing policies to manage the ethical implications of AI use in healthcare. Embracing these changes will be crucial for healthcare providers looking to improve efficiency, reduce operational costs, and enhance patient care through innovative technology.
The long-term impacts of AI in healthcare are poised to be transformative. With such systems, the efficiency of medical documentation and data management will see sustained improvement, reducing the time healthcare providers spend on paperwork and increasing their availability for patient care. This shift will likely lead to better patient engagement and satisfaction, as providers can focus more on treatment than administrative tasks. Furthermore, the precision of AI in processing and analyzing medical data means that the potential for diagnostic and treatment errors will decrease, significantly improving patient safety.